Research
In my Ph.D. study, I devote myself to Trustworthy AI research and mainly focus on the physical adversarial examples generation, now I try to transfer to investigate the adversarial defense works in the physical world. I hold the review that physical adversarial attacks and defenses can powerfully promote the development of secure and robust artificial intelligence, leading to a healthier future society. Our previous works such as bias-based universal adversarial patch attack and dual attention suppression attack have achieved some results and draw some interesting conclusions.
Now my research focus is mainly on:
-
Physical adversarial examples generation
-
Defend adversarial attacks in the physical world
-
3D adversarial attack
-
Model robustness evaluation and testing
-
Secure and trustworthy artificial intelligence
|
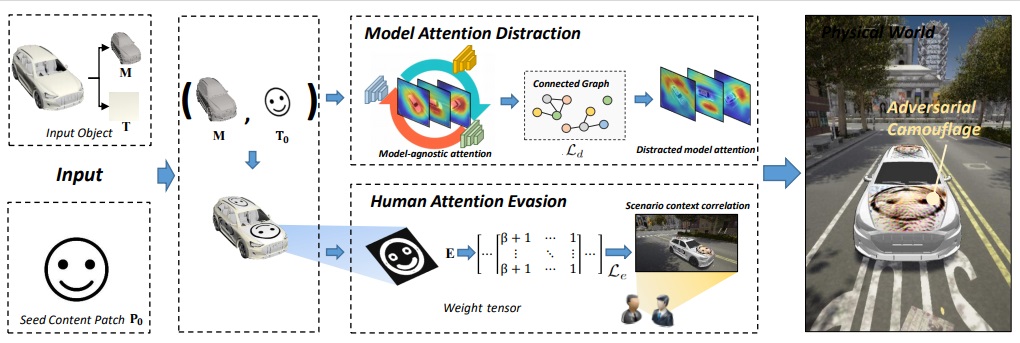 |
Dual Attention Suppression Attack: Generate Adversarial Camouflage in Physical World
Jiakai Wang, Aishan Liu, Zixin Yin, Shunchang Liu, Shiyu Tang, Xianglong Liu.
IEEE Conference on Computer Vision and Pattern Recognition (CVPR), 2021
(Oral)
pdf /
News:
(机器之心)
/Project page
We propose the Dual Attention Suppression (DAS) attack to generate visually-natural physical adversarial camouflages with strong transferability by suppressing both model and human attention.
|
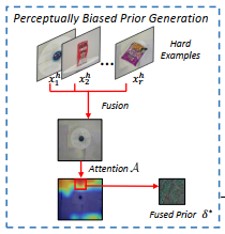 |
Bias-based Universal Adversarial Patch Attack for Automatic Check-out
Aishan Liu*, Jiakai Wang*, Xianglong Liu, Bowen Cao, Chongzhi Zhang, Hang Yu.
European Conference on Computer Vision (ECCV), 2020
pdf /
News:
(新智元)
/Project page
We propose a bias-based framework to generate class-agnostic universal adversarial patches with strong generalization ability, which exploits both the perceptual and semantic bias of models.
|
 |
Sequential Alignment Attention Model for Scene Text Recognition.
[PDF]
Yan Wu, Jiaxin Fan, Renshuai Tao*, Jiakai Wang, Haotong Qin, Aishan Liu, Xianglong Liu. (* indicates the corresponding author)
ELSEVIER Journal of Visual Communication and Image Representation (JVCI) (SCI, Q2), 2021
arXiv /
Code
In this paper, we proposes a sequential alignment attention model to enhance the alignment between input images and output character sequences.
|
 |
Towards Real-world X-ray Security Inspection: A High-Quality Benchmark And Lateral Inhibition Module For Prohibited Items Detection.
[PDF]
Renshuai Tao, Yanlu Wei, Xiangjian Jiang, Hainan Li, Haotong Qin, Jiakai Wang, Yuqing Ma, Libo Zhang, Xianglong Liu.
IEEE International Conference on Computer Vision (ICCV), CCF-A, 2021
arXiv /
Code
We present a High-quality X-ray (HiXray) security inspection image dataset and the Lateral Inhibition Module (LIM).
|
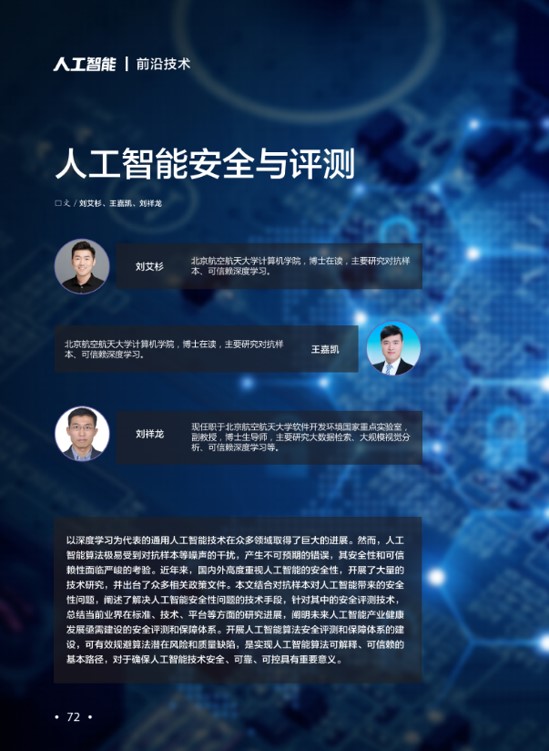 |
人工智能安全与评测
刘艾杉, 王嘉凯, 刘祥龙
人工智能(AI-View), 2020
pdf
|
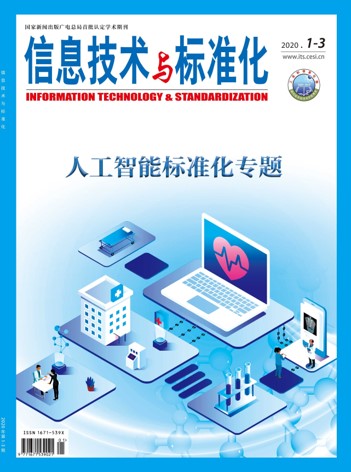 |
人工智能机器学习模型及系统的质量要素和测试方法
王嘉凯, 刘艾杉, 刘祥龙
信息技术与标准化, 2020
pdf
|
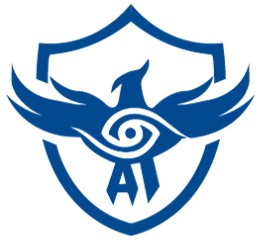 |
重明 (AISafety)
pdf /
(News: TechWeb) /
Project page
重明 is an open-source platform to evaluate model robustness and safety towards noises (e.g., adversarial examples, corruptions, etc.).
The name is taken from the Chinese myth 重明鸟, which has strong power, could fight against beasts and avoid disasters.
We hope our platform could improve the robustness of deep learning systems and help them to avoid safety-related problems.
重明 has been awarded the 首届OpenI启智社区优秀开源项目 (First OpenI Excellent Open Source Project).
|
Main Awards
[2021.06] Beihang University Excellent Academic Paper Award.
[2020.10] Beihang University First Prize Scholarship.
[2020.09] China National Scholarship (Top2%).
[2020.09] Beihang University Merit Student.
[2019.10] Beihang University First Prize Scholarship.
[2018.09] Beihang University Outstanding Freshman Scholarship (1/12).
[2018.06] Outstanding Graduates of Beijing Province.
|
|